Introduction
In the age of artificial intelligence advancement, it is not an unpredictable future to see autonomous vehicles driven(!) in our streets. In 2015, the Guardian reported that front seat drivers would become backseat drivers permanently by 2020 (Adams, 2015). This prediction was accompanied by the declarations from several self-driving car manufacturers (General Motors, Google, and Tesla). Although extraordinary efforts have been given by these leading tech companies to finalise the proper function, the current state of affair of self-driving cars does not materalise the target yet, except in special trial programs, e.g. Tesla Model S Autopilot which controls highway driving. Amongst others, such as technical development and availability of the infrastructure, one of the reasons behind the delay is the ethical concerns associated with self-driving cars.
The impact of ethics in self-driving cars is a controversial topic, and any approach to the discussion is shaped by subjective factors such as cultural background, perception of values, philosophical tendencies. The main focus of any ethical discussion about the matter is given to the situation where a self-driving car involves in a traffic accident. Two ethical concerns have arisen in such an incident. The first concern addresses an ex-post analysis of the incident and seeks the identification of the person or entity accountable for the crash. In other words, it is the question of who to blame; whether vehicle manufacturers, automated driving system developers, data providers, owners of the vehicle or other road users who play a role in bringing about the crash. The second concern addresses an ex-ante analysis and questions the decision-making procedure of self-driving cars in each surrounding circumstance. For instance, whether a self-driving car should be instructed to hit a car in the front lane or hit an old pedestrian in another lane by changing its route when an accident is inevitable. In that regard, this ethical concern signals the similarity with the ethical dilemma illustrated with the trolley problem.
Self-Driving Cars
Equipping cars with recent technological developments will be likely to reduce crashes, pollution and energy consumption. Therefore, the main reason behind the automation is the willingness to increase the safety, reliability, and efficiency of the cars through the fixed standardisation of system operation with limited or without human intervention. When using the concept of âautomationâ with respect to car production, the literature conceptualises the term by categorising it with the levels suggested by the US National Highway Traffic Safety Administration (NHTSA) as can be seen in Figure No.1 (Wetzel, 2020).
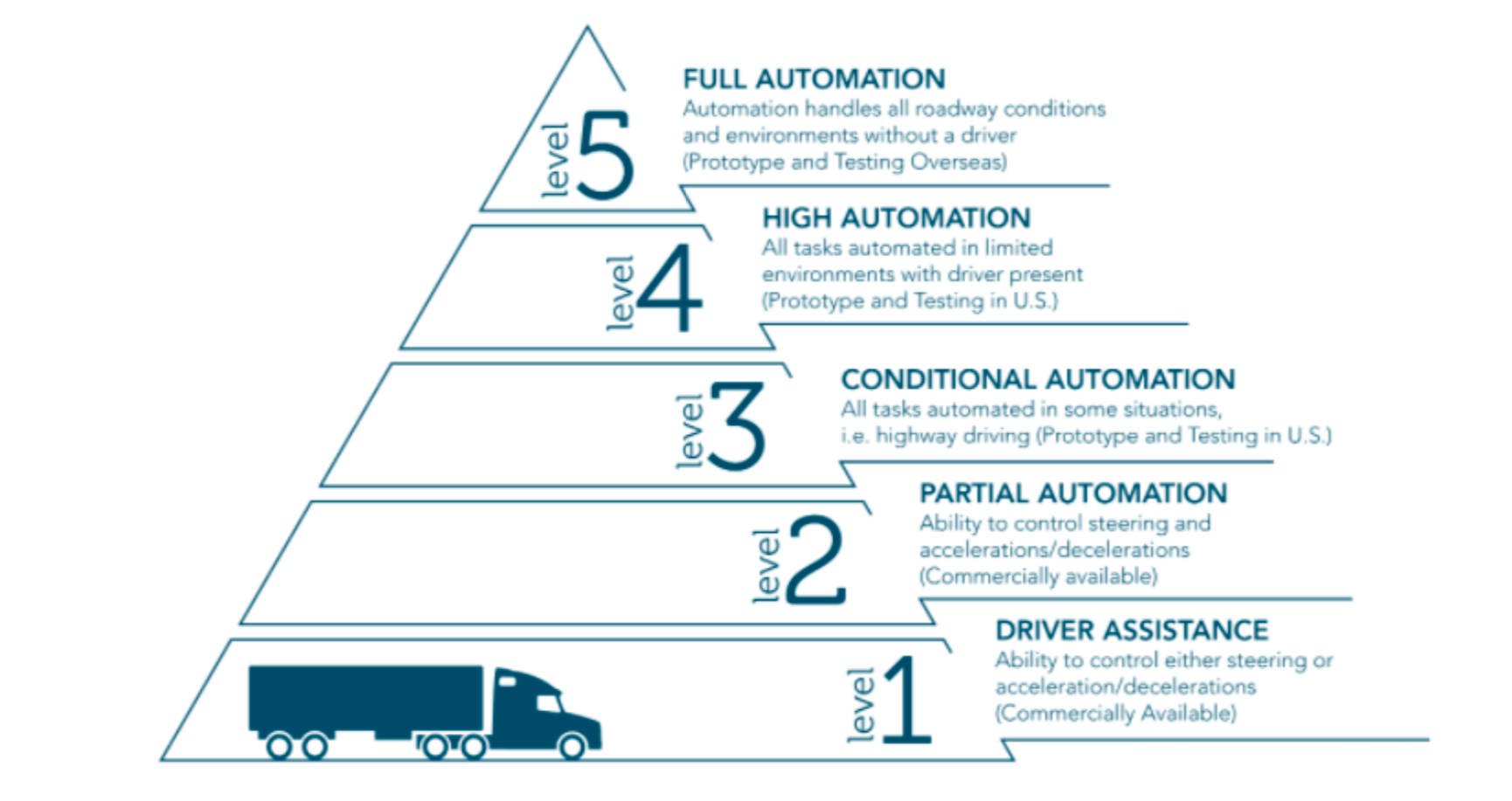
The first ethical concern, namely the accountability in the case of a traffic accident, is getting more sophisticated from the first level, where there is a full driver responsibility with strict liability, to higher levels because of the gradual control loss. The second ethical concern, namely the decision-making procedure of self-driving cars, became the primary dilemma starting from level three upwards, where the automation begins to function as a decision-maker (limited in level three but full control in level five), which would have been done by human-beings in normal circumstances.
The Trolley Problem
When addressing the ethical concern caused by self-driving cars, the literature always references to the trolley problem (Maurer et al. 2015, p.78; Lin, Abney and Jenkins, 2017, p. 23). This moral paradox was first mentioned by Philippa Foot in 1967 and asked what a bystander should do in a scenario where an uncontrolled trolley is hurtling down toward five people tied up to railroad tracks (Foot, 2002). This bystander can access a lever which enables him to divert the trolley to the second alternative track where there is only one person tied up. This catastrophic incident constitutes a dilemma for the bystander to determine either watching the death of five people by choosing a passive behaviour or causing the death of one individual by actively intervening in the situation and saving the other five.
Once a self-driving car is on the road (representing the trolley), the lever panels are previously determined by manufacturers, particularly by programmers, who act as bystanders by assessing all hypothetical scenarios in advance (Marshall, 2018). The problem with the self-driving car is that there is no passive outcome, unlike the trolley problem, because each alternative is programmed at the stage of manufacturing. In other words, every outcome is programmed as a lever panel; whether the car should stay in the lane and cause injury (or even death) of passengers or swerve to the group of pedestrians and save the passengers, whether the amount, gender, age, physical appearance or occupation of the pedestrians or passengers matter or not (Goodall, 2016). It would be naĂŻve to think that we would not need such an accident-algorithm as if Nyholm drew an analogy of Titanic that would not carry life-boats because it was an âunsinkableâ ship (Nyholm, 2018, p.2). As a result, although these situations are unlikely to arise, self-driving carsâ programming must cover decision rules about what to do in hypothetical scenarios. To do so, Bonnefon and Rahwan claim that algorithms should be trained with moral principles that shape the decision-making procedure in case harm is unavoidable (Bonnefon et al., 2016).
MIT Moral Machine
It is not easy to produce globally accepted moral principles to resolve the ethical dilemma because what is the right thing to do may be evaluated, interpreted and assessed differently. Due to this potential inconsistency, researchers aim to find the most tolerable preferences by organizing the data-driven study of driverless car ethics. In that regard, MIT Media Lab researchers designed an experiment, Moral Machine, that simulates hypothetical scenarios and crowdsources internet usersâ decisions on how self-driving cars should behave in each scenario having different variations of the trolley problem (Awad et al., 2018).
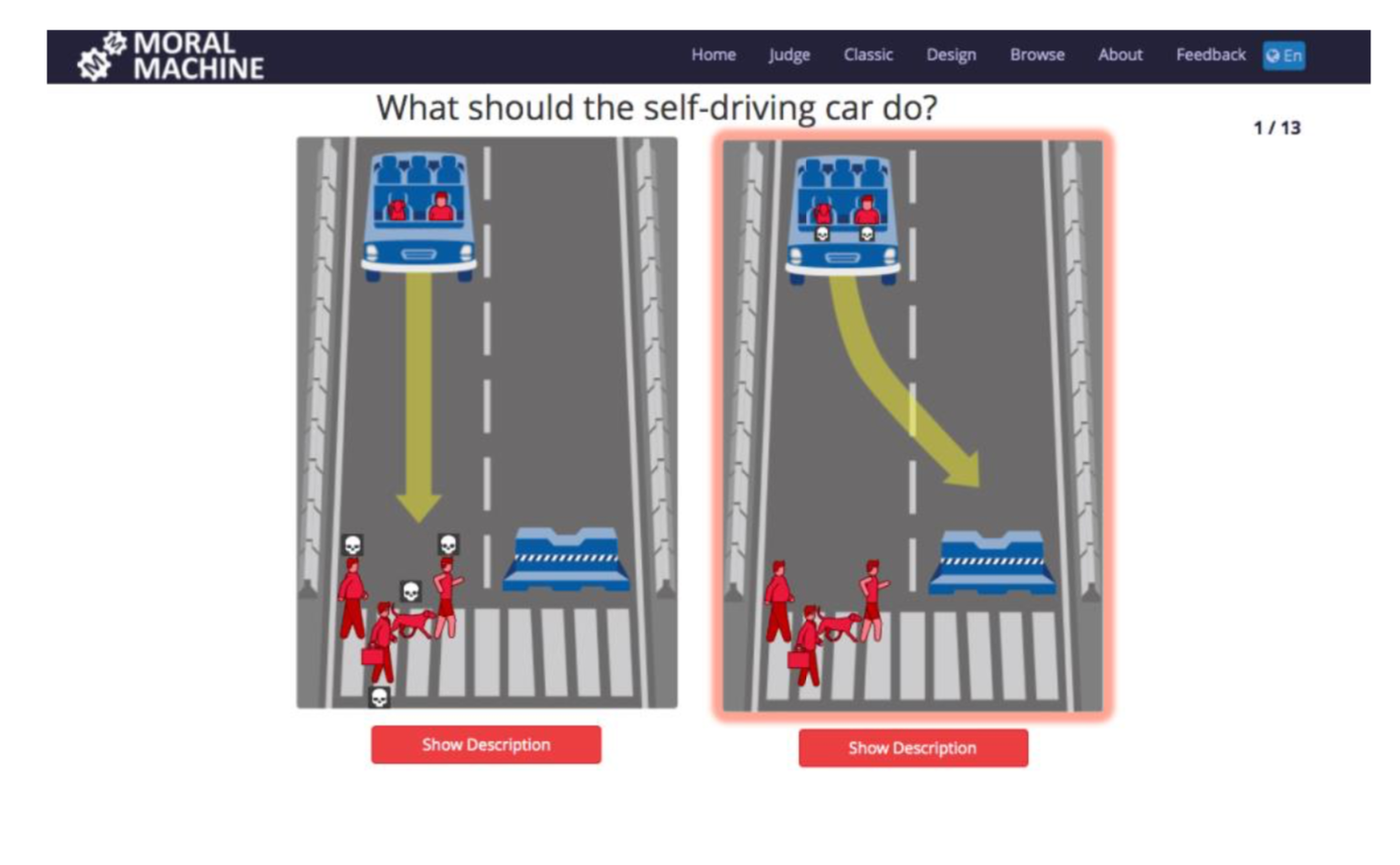
This online game collects large-scale data on how individuals would prefer self-driving cars to solve moral dilemmas in a situation where an accident is unavoidable. The platform provides two possible outcomes of which a user needs to decide whether the self-driving car swerves or remains staying on the lane. The characters within the simulation vary depending on several factors such as the gender, age, social status, occupation, physical appearance, whether a person is a pedestrian or passenger, whether (s)he crosses legally or jaywalks.
The experiment has an empirical value that illustrates the globally preferable moral judgements by establishing patterns observed from the preferences in hypothetical scenarios. The platform documents the choices based on the respondentsâ demographic information such as country of residence, age, gender, income, political and religious views. Considering the data-scale and content of the platform, the experiment has the potential to contribute to the development of ethical principles of self-driving cars by taking into account socially tolerable preferences (Barker, 2017). For instance, there are strong preferences to save humans rather than animals, more lives rather than the less amount, and young lives over the elders (Awad et al., 2018, p.60). Therefore, these preferences can be considered as the building blocks for programming self-driving cars, or at least constitute an indicator material for policymakers to consider. However, when it comes to the regulation of these preferences, Rahwan - a researcher affiliated with Moral Machine - welcomes opposing and controversial opinions as there is no universal set of rules for robotics (Maxmen, 2018). For instance, the German Ethics Commission on Automated and Connected Driving produced a guideline in 2017 regarding the ethical principles governing self-driving cars (Germany. Federal Ministry of Transport and Digital Infrastructure, 2017). Accordingly, there is an overlap with regards to sparing humans over animals and reducing the number personal injuries that the Guideline priorities the enjoyment of human life at the highest, similar to the social expectations illustrated by the Moral Machine (Principle 7). However, the Guideline prohibits any distinction based on characteristic features such as age, gender, physical or mental constitution (Principle 9), which contradicts with the strong preferences of saving young generations over the elder ones observed through the Moral Machine. Such a contradiction does not mean that policymakers are obliged to prefer the majoritarian public opinion observed in the experiment, nevertheless, disapplying the public opinion may pose challenges for policymakers in justifying the rationale of their decision, hence may create a strong backlash from society.
Criticism to the Trolley Problem
At first sight, the dilemma faced by the bystander in the trolley problem and its application via the Moral Machine shows successful resemblance with the ethical concern that self-driving car programmers involve in. However, this analogy has the risk of not fully reflecting the ethical concern of self-driving cars. In fact, the analogy has been challenged by the literature for three reasons (Hubner & White, 2018; Nyholm. 2018).
First, the analogy and the virtual experiment enable deepening the discussion over the ethical concern; however, they do not provide an agreed ethical consensus that solves the problem of programming the algorithms in the event of a crash. Professor Lin claims that the trolley problem successfully illustrates the incongruities between ethical theories, rather than providing a solution to the ethical concern (Lin, 2017, p. 79). The utilitarian theory, based on the ideas of Jeremy Bentham, would justify switching the lever panel in the trolley problem (hence prioritises the death of one person rather than five people) because the theory focuses on the outcome, and seeks for maximum happiness while minimising overall suffering (Nyholm. 2018). On the other hand, a deontologist, based on the ideas of Kantâs categorical imperative, would not switch the lever panel (hence prioritises the death of five people rather than one person by relying on the nature of the act) because the theory follows a set of absolute moral principles in any circumstances, irrespective of the outcome (Gurney, 2015). Gogoll and Muller also underline that adopting one of these theories would contradict with the standards of a liberal society by obliging a person to act in a certain way although (s)he would not freely agree (Gogoll & Muller, 2017; Loh & Loh, 2017, pp. 35-50). Therefore, the trolley problem has resulted in illustrating the controversy of competing outlooks; however, none of these theories can be chosen to govern the self-driving carsâ ethical concern by itself because they can be reasonably rejected.
Second, Gogoll and Muller draw out that the trolley problem only focuses on the viewpoint of a third-party who is not affected by the traffic crash and disregards the perspectives of people affected by accident such as drivers or other passengers (Gogoll & Muller, 2017). It would be likely to expect from Moral Machine respondents to decide completely opposite direction if they were involved in a similar scenario with themselves, rather than judging as third-party. Therefore, for instance, the data held by the Moral Machine may have the risk of bias by consisting of information that does not reflect the actual and practical choice of the respondents.
Thirdly, the analogy does not fully reflect a traffic crash in real-life. The trolley problem provides an abstract and deterministic scenario that excludes the probabilities because the outcomes of the given options are certain, namely death. However, Nyholm and Smids point out that, in real life, it would not be possible to ascertain the outcome; instead a risk-assessment is being made between either side (Nyholm & Smids, 2016). When self-driving cars are coded, it deals with probable outcomes, e.g. if A is coded rather than B, it is X percent less likely to hit a pedestrian. Therefore, the analogy is criticised due to its simplification of the choice situation by compelling the fatal outcome.
Policy Considerations
- Ethical Settings Tool
The trolley problem has successfully illustrated the discussion between ethical theories; however, it does not provide a final solution on how to program accident-algorithms due to the lack of universal consensus on choosing one governing theory. Therefore, manufacturers cannot adopt and justify a single ethical theory to program accident-algorithms. Alternatively, some scholars argue that self-driving cars should function with the ethical perspective of its owner (Contissa, Lagioia & Sartor, 2017). Accordingly, it is suggested that self-driving cars should be equipped with an ethical settings tool that enables its users to customise their preferred ethical theories of which they found proximity. By providing the users with a degree of choice, the suggestion also resolves the issues with accountability because it would be easier to hold the users responsible for the outcome of a traffic crash. However, this proposition is strongly objected by Professor Lin who underlines that the possibility of choosing their own ethical settings can be unacceptably used by some people having racist ideologies or other unacceptable perspectives (Maurer et al. 2015, p.80). Therefore, it is one of the future considerations for policymakers to balance the possibility of implementing an ethical settings tool with the view that everyone should use the same ethical setting.
2. Geographical Implementation of Choice
Since it is difficult to reach out using the same ethical theory universally, relatively narrower boundaries, such as demographic preferences, can be drawn to apply the same ethical settings. For instance, according to the Moral Machine data, the majority of Latin American respondents are willing to save the younger generation rather than the older one, which is less pronounced by Eastern respondents (Awad et al., 2018, p.61). Therefore, accident-algorithms can be programmed in accordance with the expectations of certain geographic levels, which can be supplemented with the use of virtual experimentsâ data, such as the Moral Machine. However, policymakers should take into account that experimentsâ data have the risk of not reflecting the actual individual preferences as respondents were asked to judge the given scenarios as being unaffected third parties. Furthermore, geographically applicable machine ethics would also create unanswered questions; for instance, which ethical preferences should Latin American-licensed self-driving cars follow while travelling in Eastern territories? How will geographically different pre-set accident-algorithms affect the legal responsibility of people involved in a traffic crash? Would it create legal uncertainty? Thus, while limiting the application of the same ethical theory, policymakers should have a careful consideration to not leave any question unanswered.
3. Privacy Consideration
Giving a high focus on accident-algorithms overshadows other ethical concerns surrounding self-driving cars, such as privacy considerations. In order for self-driving cars to work, the technology behind stores an ocean of data that, for instance, a single car should know the exact destination and targeted destination and should track anything on the road by using high-tech cameras and GPS navigation. Therefore, self-driving cars will also collect and store personal information that owners would not know how this information is being used, which inherently signals privacy-related ethical concerns (Nyholm, 2018, p.9). Besides, the more personalised these self-driving cars, the more vulnerable they are in terms of the risk of privacy infringement. For instance, in case an ethical setting tool is incorporated with the design as suggested by the scholars above, it would constitute highly sensitive and personalised information about individualsâ ethical tendencies, and any privacy infringement would create severe consequences. Besides, the aggregated data gathered from self-driving cars have the potential value for companies to monetise by means of marketing channels. For example, a manufacturer can easily detect the transportation habits (by analysing the patterns from someoneâs locations, preferences and behaviours) of self- driving carsâ owners. This can be used by insurance companies while determining insurance premiums or by third parties who can target the owners with location-based advertisements. This is not a far-fetched scenario because there is already a vehicle data monetisation market worth $2 billion that has the potential to increase to $33 billion in a decade (Hedlund, 2019). Therefore, policymakers should not neglect other ethical concerns by overwhelmingly focusing on the dilemma of accident-algorithms for the sake of the protection of general consumer information and considers cybersecurity plans by evaluating the vulnerabilities of self-driving cars.
Conclusion
It is the first time that Asimovâs rules on robotics, namely a robot may not injure a human being, may be overruled because self-driving cars will be programmed with making ethical decisions when confronted with an unavoidable traffic crash. Hence, before manufacturers design self-driving cars to make a moral judgement, we need to establish a global platform to discuss the ethical concern on a broad scale and policymakers must be involve in regulating the issue. For this, we need to ask the correct questions and the trolley problem can be useful in that regard. However, the trolley problem does not provide answers to the questions it poses, hence can only be used as a starting point to the global conversation. Further, it can lead to derivative practice-related questions for policymakers to consider while evaluating the ethical concerns on self-driving cars.